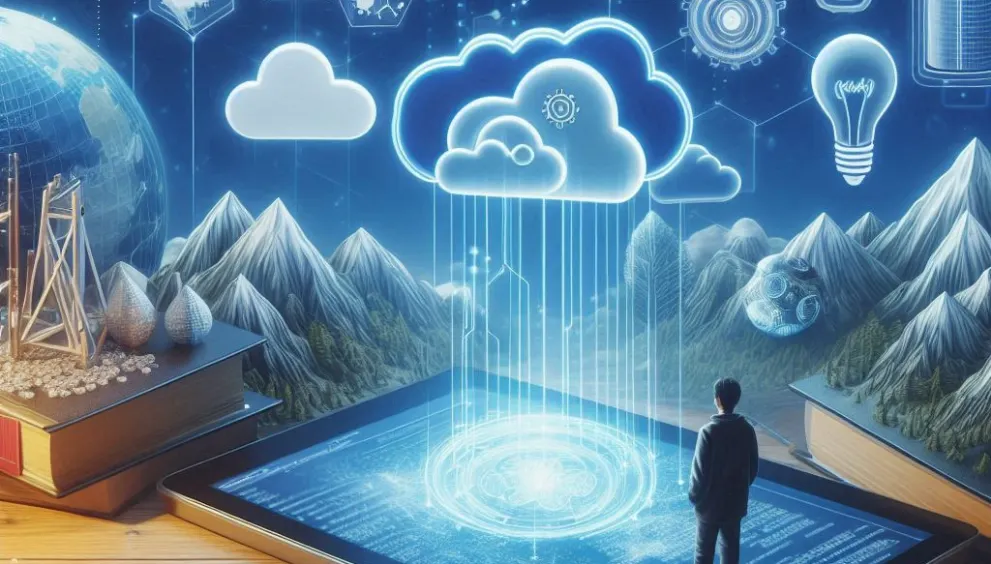
From this to……................................................this
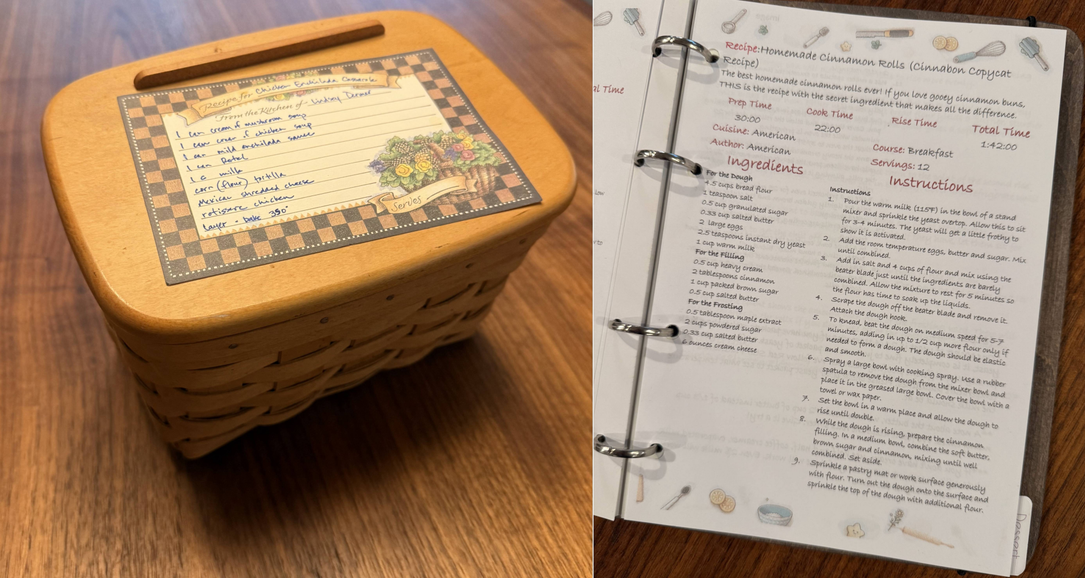
using Azure OpenAI REST and .NET Azure.AI.OpenAI SDK.
Let me start off with a big thanks to the contributors at OpenAI gpt4 vision pdf extraction sample . I greatly appreciate them sharing their knowledge.
Now on too to a practical personal requirement and thinking about it in terms of a deployable business AI workflow in an azure ai production environment. I have diagramed the vision of how I think it will best be deployed in an Azure environment. As I start to prototype type out the solution, I’ll share insights along the way as each component gets built out and likely the initial diagram gets updated taking into account learnings along the way.
The Vision
The Requirement
With a multitude of recipes sitting on the shelf in folders, scratch papers, and index card holders I wanted an automated method to transfer them all to a standard format and into a recent recipe book I received for Christmas last year.
My requirements:
- Ability to drop an image or pdf into a folder or album and have it automatically produce a word document from a word template.
- Ability to pull up the original document and compare to the word document in order to make any manual adjustments.
- Use an AI model and have the ability to compare different models for cost, quality, and ease of use.
- Set up the overall architecture and environment simulating a production type scenario using the process as a learning tool for implementing all the various best practices.
- Longer term determine if there is a device deployable model that could handle the work vs. setting up an Azure environment. IE could all this run on my iPhone (likely, but let’s do some fun stuff first).
The Solution
My first draft of the envisioned solution. The two key pieces are an azure function that takes an image of a recipe and converts it to json. The 2nd is a json to word function. The rest is all the infrastructure around setting this up in an Azure production like flow. Getting photos from an album to onedrive or Azure may be the hardest part.
Side note: read here for a shortcut to taking pictures of your recipe index cards.
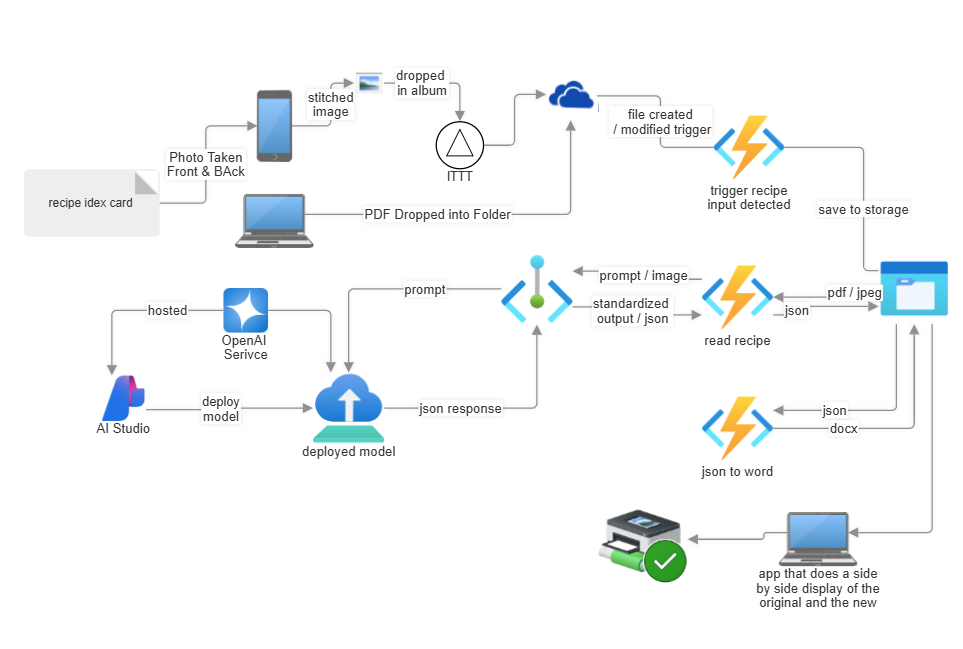
Key Learnings
In case you want to skip all the reading
- Models that don’t support standardized_output you pass in either a json schema or an example json document that represents what you want to come out of the model.
- If content filters are triggered you still get a 200, your json is truncated and the response includes a json section that defines the content filtering results. Note: this is what I observed with the Azure OpenAI hosted models. Be sure and look at the returned response and parse through the content filter sections.
- If the model does not support image input an error something like : Error copying to stream is returned.
- structured_output – way cool for the models that support it, if you have a class that you want the model to return the answer in use the CreateJsonSchemaFormat with your class. Not that many models currently support structured output.
- ChatCompletionOptions options = new ChatCompletionOptions()
{
ResponseFormat = StructuredOutputsExtensions.CreateJsonSchemaFormat<Recipe>(“Recipe”, jsonSchemaIsStrict: true),
MaxOutputTokenCount = 14000,
Temperature = 0.1f,
TopP = 1.0f,
};
- ChatCompletionOptions options = new ChatCompletionOptions()
Prototype
To prototype out the two key methods I developed two console apps. One that reads all the pdf and jpeg files in an input directory and calls the AI Engine to get the json for the recipe and save’s the json in a folder. Another console app that takes the json and reads a word document used as a template and merges in the json recipe information into the word document. In an enterprise environment a software reporting product would likely be used vs a custom app, but yeah it’s another learning opportunity.
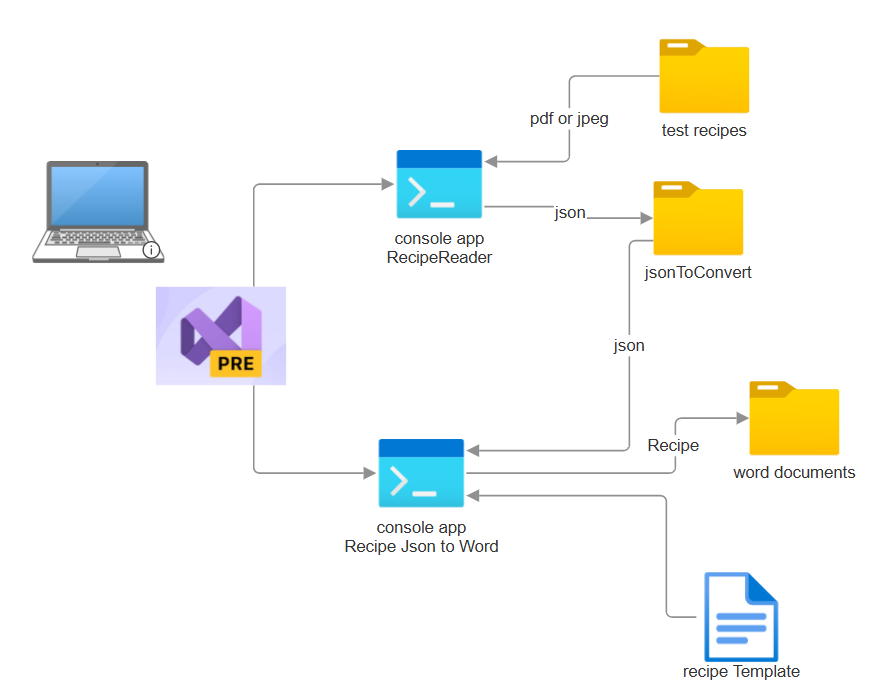
implements two different methods for a calling the AI engine. A REST based method and a method using the Azure.AI.OpenAI library.
The basic flow is
- get configuration information from file
- Get list of files
- foreach file
- convert pdf to image, or read image file
- craft prompt
- call the AI Services
- parse return and save the json in a file
I set up the app to read a .env file so that I could easily configure different end points and models to run through either the REST method or the SDK method. The program can also take individual parameters for each option. The first three environment variables come from your Azure OpenAI configuration. I’ll walk through that later in the article. Having multiple configuration files let’s me easily test different models and OpenAI configurations of those models.
1 2 3 4 5 | AI_ENDPOINT=https://ai-xxxxx.openai.azure.com/ MODEL_DEPLOYMENT=gpt-4oRecipeReader API_VERSION=2024-08-01-preview RECIPE_DIRECTORY=C:\Users\brady\OneDrive\root\08-RecipeToWordViaAI\test recipes OUTPUT_DIRECTORY=C:\Users\brady\OneDrive\root\08-RecipeToWordViaAI\test recipes\output |
As mentioned earlier I developed two different methods for calling the model inference engine? A REST based method and using the OpenAI SDK. I started with the REST because that’s what the sample I started with used. I quickly found the SDK and wanted to see if there was much of a difference. I’ll start with the REST method.
REST Method
The actual call to the AI engine is not all the complex. It’s a typical PoastAsync. Formatting the input is where the work is done.
1 2 3 4 5 6 7 8 9 10 11 12 13 14 15 16 17 18 19 20 21 22 23 24 25 26 27 28 29 30 31 32 33 34 35 36 37 38 39 40 41 42 43 44 45 46 47 48 49 50 51 52 53 54 55 56 57 58 59 60 61 62 63 64 65 66 67 68 69 70 71 72 73 74 | using System.Collections.Generic; using System.Linq; using System.Net.Http; using System.Text; using System.Threading.Tasks; using Azure.Core; using Azure; using Azure.AI.OpenAI; using Azure.Identity; using DotNetEnv; using System.Diagnostics; using MyNextBook.Helpers; using RecipeReader.Helpers; using Newtonsoft.Json.Linq; namespace RecipeReader { static public class AIServicesREST { static async public Task<HttpResponseMessage> ExtractRecipeFromPDFAsync(string payload, string? endPoint, string? modelDeployment, string? apiVersion) { JObject j = JObject.Parse(payload); JsonHelper.PrintKeyValuePairs(j); DefaultAzureCredential? credential; credential = new DefaultAzureCredential(new DefaultAzureCredentialOptions { ExcludeEnvironmentCredential = true, ExcludeManagedIdentityCredential = true, ExcludeSharedTokenCacheCredential = true, ExcludeInteractiveBrowserCredential = true, ExcludeAzurePowerShellCredential = true, ExcludeVisualStudioCodeCredential = false, ExcludeAzureCliCredential = false }); var bearerToken = credential.GetToken(new TokenRequestContext(new[] { "https://cognitiveservices.azure.com/.default" })).Token; using (HttpClient httpClient = new HttpClient { Timeout = TimeSpan.FromMinutes(10) }) { HttpResponseMessage? response = null; string fullEndPoint = $"{endPoint}openai/deployments/{modelDeployment}/chat/completions?api-version={apiVersion}"; httpClient.BaseAddress = new Uri(fullEndPoint); ErrorHandler.AddLog("Using AI at: " + fullEndPoint); httpClient.DefaultRequestHeaders.Add("Authorization", $"Bearer {bearerToken}"); httpClient.DefaultRequestHeaders.Accept.Add(new System.Net.Http.Headers.MediaTypeWithQualityHeaderValue("application/json")); var stringContent = new StringContent(payload, Encoding.UTF8, "application/json"); try { response = await httpClient.PostAsync(fullEndPoint, stringContent); } catch (Exception ex) { ErrorHandler.AddError(ex); ErrorHandler.AddLog(ex.Message); ErrorHandler.AddLog(ex.InnerException?.Message); } return response; } } } } |
The payload to the post is a JsonObject that is an array of messages and parameters for OpenAI to use. Here you can see that the messages provide the input to the chat for completion using the role syntax. “System” : “you are a…” “user” : “Extract the recipe”…
Note that the recipe image is part of the message as a base64 string. The message type is : “type” , “image_url”.
Additionally notice on line 18 that the struction of the requested json is passed in as part of the prompt. The exampleRecipeJson is a json format of the Recipe object and is provided as part of the prompt. I had one of the AI engines generate an example json recipe that had all the structured components I was looking for and used it as an example and used it to create the initial class structure for a Recipe.
1 2 3 4 5 6 7 8 9 10 11 12 13 14 15 16 17 18 19 20 21 22 23 24 25 26 27 28 29 30 31 32 33 34 35 36 37 38 39 40 41 42 43 44 45 46 47 48 49 50 51 | ....................... In a help method the pdf is converted to an image and the image is converted to base64 and inserted into jsonObject. var jsonObject = new JsonObject { { "type", "image_url" }, { "image_url", new JsonObject { { "url", $"data:image/jpeg;base64,{base64Image}" } } } }; ................... var userPromptParts = new List<JsonNode>{ new JsonObject { { "type", "text" }, { "text", $"Extract the recipe from this document. Note that notes are often multiple paragraphs be sure to capture all the notes." + "If a value is not present, provide null Use the following" + $"structure:{exampleRecipeJson}" } }}; userPromptParts.AddRange(recipeImageData); JsonObject jsonPayload = new JsonObject { { "messages", new JsonArray { new JsonObject { { "role", "system" }, { "content", "You are an AI assistant that extracts recipe instructions and ingredients from documents and returns them as structured JSON objects. including copyright and author information in the response Do not return as a code block." } }, new JsonObject { { "role", "user" }, { "content", new JsonArray(userPromptParts.ToArray())} } } }, { "model", modelDeployment }, { "max_tokens", 8096 }, { "temperature", 0.1 }, { "top_p", 0.01 } }; string payload = JsonSerializer.Serialize(jsonPayload, new JsonSerializerOptions { WriteIndented = true }); |
I had the program output the input parameters and the result along with some processing information to a log file. The following output is from a run using gpt4o. At the end of the log you can see the number of tokens input output and total. And the processing time is a stopwatch in the program that starts/ends before and after the call.
1 2 3 4 5 6 7 8 9 10 11 12 13 14 15 16 17 18 19 20 21 22 23 24 25 26 27 28 29 30 31 32 33 34 35 36 37 38 39 40 41 42 43 44 45 46 47 48 49 50 51 52 53 54 55 56 57 58 59 60 61 62 63 64 65 66 67 68 69 70 71 72 73 74 75 76 77 78 79 80 81 82 83 84 85 86 87 88 89 90 91 92 93 94 95 96 97 98 99 100 101 102 103 104 105 106 107 108 109 110 111 112 113 114 115 116 117 118 119 120 121 122 123 124 125 126 127 128 129 130 131 132 133 134 135 136 137 138 139 140 141 142 143 144 145 146 147 148 149 150 151 152 153 154 155 156 157 158 159 160 161 162 163 164 165 166 167 168 169 170 171 172 173 174 175 176 177 178 179 180 181 182 183 184 185 186 187 188 189 190 191 192 193 194 195 196 197 198 199 200 201 202 203 204 205 206 207 208 209 210 211 212 213 214 215 216 217 218 219 220 221 222 223 224 225 226 227 228 229 230 231 232 233 234 235 236 237 238 239 240 241 242 243 244 245 246 247 248 249 250 251 252 253 254 255 256 257 258 259 260 261 262 263 264 265 266 267 268 269 270 271 272 273 274 275 276 277 278 279 280 281 282 283 284 285 286 287 288 289 290 291 292 293 294 295 296 297 298 299 300 301 302 303 304 305 306 307 308 309 310 311 312 313 314 315 316 317 318 319 320 321 322 323 324 325 326 327 328 329 330 331 332 333 334 335 336 337 338 339 340 341 342 343 344 345 346 347 348 349 350 351 352 353 354 355 356 357 358 359 360 361 362 363 364 365 366 367 368 369 370 371 372 373 374 375 376 377 378 379 380 381 382 383 384 385 386 387 388 389 390 391 392 393 394 395 396 397 398 399 400 401 402 403 404 | Starting Recipe Reader... Model: gpt-4oRecipeReader API Version: 2024-08-01-preview AI Endpoint: https://ai-recipereader.openai.azure.com/ Processing recipes from: C:\Users\brady\OneDrive\root\08-RecipeToWordViaAI\test recipes Outputting parsed recipes to: C:\Users\brady\OneDrive\root\08-RecipeToWordViaAI\test recipes\output Number of files in directory: 1 Using REST Method messages: [ role: system content: You are an AI assistant that extracts recipe instructions and ingredients from documents and returns them as structured JSON objects. including copyright and author information in the response Do not return as a code block. role: user content: [ type: text text: Extract the recipe from this document. Note that notes are often multiple paragraphs be sure to capture all the notes.If a value is not present, provide null Use the followingstructure:{ "id": 1, "name": "Grilled Chicken Fajitas", "description": "Sizzling fajitas with marinated chicken, bell peppers, onions, and warm flour tortillas.", "servings": 4, "cookTime": "20:00", "prepTime": "15:00", "totalTime": "35:00", "author": "John Doe", "cuisine": "Mexican", "course": "Main Course", "image": "https://example.com/grilled-chicken-fajitas.jpg", "calories": 420, "nutrition": { "protein": 35, "fat": 20, "carbohydrates": 25, "fiber": 5, "sugar": 5, "sodium": 350 }, "ingredientGroups": [ { "name": "Proteins", "ingredients": [ { "name": "boneless, skinless chicken breasts", "quantity": 1.5, "unit": "pounds" } ] }, { "name": "Produce", "ingredients": [ { "name": "bell peppers", "quantity": 2, "unit": "medium" }, { "name": "onion", "quantity": 1, "unit": "large" } ] }, { "name": "Pantry", "ingredients": [ { "name": "flour tortillas", "quantity": 8, "unit": "tortillas" }, { "name": "fajita seasoning", "quantity": 1, "unit": "packet" } ] } ], "instructionGroups": [ { "name": "Preparation", "instructions": [ { "step": 1, "description": "Preheat grill to medium-high heat." }, { "step": 2, "description": "Marinate chicken in fajita seasoning for at least 30 minutes." } ] }, { "name": "Grilling", "instructions": [ { "step": 3, "description": "Grill chicken for 5-6 minutes per side, or until cooked through." }, { "step": 4, "description": "Grill bell peppers and onion for 3-4 minutes per side, or until tender." } ] }, { "name": "Assembly", "instructions": [ { "step": 5, "description": "Warm flour tortillas by wrapping them in foil and heating them on the grill for 2-3 minutes." }, { "step": 6, "description": "Assemble fajitas by slicing chicken and vegetables, and serving with warm tortillas." } ] } ], "tags": ["Mexican", "grilled", "chicken", "fajitas"], "notes": "You can also add other toppings like sour cream, salsa, avocado, and shredded cheese to make it more delicious." } type: image_url image_url: url: data:image/jpeg;base64,/9j/4AA... type: image_url image_url: url: data:image/jpeg;base64,/9j/4AA... ] ] model: gpt-4oRecipeReader max_tokens: 8096 temperature: 0.1 top_p: 0.01 Using AI at: https://ai-recipereader.openai.azure.com/openai/deployments/gpt-4oRecipeReader/chat/completions?api-version=2024-08-01-preview choices: [ content_filter_results: hate: filtered: False severity: safe self_harm: filtered: False severity: safe sexual: filtered: False severity: safe violence: filtered: False severity: safe finish_reason: stop index: 0 logprobs: message: content: { "id": 1, "name": "Rocky Road Fudge Bars", "description": null, "servings": null, "cookTime": null, "prepTime": null, "totalTime": null, "author": null, "cuisine": null, "course": null, "image": null, "calories": null, "nutrition": { "protein": null, "fat": null, "carbohydrates": null, "fiber": null, "sugar": null, "sodium": null }, "ingredientGroups": [ { "name": "Crust", "ingredients": [ { "name": "unsalted butter", "quantity": 0.5, "unit": "cup" }, { "name": "unsweetened chocolate, finely chopped", "quantity": 2, "unit": "ounces" }, { "name": "sugar", "quantity": 1, "unit": "cup" }, { "name": "all purpose flour", "quantity": 1, "unit": "cup" }, { "name": "chopped blanched almonds (optional)", "quantity": 1, "unit": "cup" }, { "name": "baking powder", "quantity": 1, "unit": "teaspoon" }, { "name": "vanilla extract", "quantity": 1, "unit": "teaspoon" } ] }, { "name": "Filling", "ingredients": [ { "name": "cream cheese", "quantity": 8, "unit": "ounces" }, { "name": "sugar", "quantity": 0.5, "unit": "cup" }, { "name": "butter, unsalted, room temperature", "quantity": 0.25, "unit": "cup" }, { "name": "all purpose flour", "quantity": 2, "unit": "tablespoons" }, { "name": "large egg", "quantity": 1, "unit": null }, { "name": "vanilla extract", "quantity": 0.5, "unit": "teaspoon" }, { "name": "chopped almonds (optional)", "quantity": 0.25, "unit": "cup" }, { "name": "semi sweet chocolate chips", "quantity": 1, "unit": "6 ounce package" }, { "name": "miniature marshmallows", "quantity": 2, "unit": "cups" } ] }, { "name": "Topping", "ingredients": [ { "name": "unsalted butter", "quantity": 0.25, "unit": "cup" }, { "name": "milk", "quantity": 0.25, "unit": "cup" }, { "name": "cream cheese", "quantity": 2, "unit": "ounces" }, { "name": "unsweetened chocolate, finely chopped", "quantity": 1, "unit": "ounce" }, { "name": "powder sugar", "quantity": 3.5, "unit": "cups" }, { "name": "vanilla extract", "quantity": 1, "unit": "teaspoon" } ] } ], "instructionGroups": [ { "name": "Crust", "instructions": [ { "step": 1, "description": "Preheat oven to 350°F. Grease and flour 9x13 inch baking dish. Melt 1/2 cup butter and chocolate in heavy sauce pan over low heat. Stir until smooth. Combine sugar, flour, almonds and baking powder in medium bowl. Add chocolate mixture and vanilla and stir until crumbly dough forms. Press into bottom of prepared pan, forming even layer." } ] }, { "name": "Filling", "instructions": [ { "step": 2, "description": "Beat first 6 ingredients together in medium bowl until fluffy. Stir in almonds. Spread evenly over dough. Sprinkle with chocolate chips. Bake until tester inserted in center comes out clean, about 30 minutes. Top with marshmallows and bake 2 minutes." } ] }, { "name": "Topping", "instructions": [ { "step": 3, "description": "Cook first four ingredients in heavy large saucepan over low heat, stirring until smooth. Add sugar and vanilla and mix until smooth. Pour over marshmallows. Swirl with knife. Let stand until topping is set. Can be prepared 2 days ahead cover tightly." } ] } ], "tags": null, "notes": null } role: assistant ] created: 1731455024 id: chatcmpl-ASupsfYIK1ug9GFLyEHVn5pYnoWd8 model: gpt-4o-2024-08-06 object: chat.completion prompt_filter_results: [ prompt_index: 0 content_filter_result: sexual: filtered: False severity: safe violence: filtered: False severity: safe hate: filtered: False severity: safe self_harm: filtered: False severity: safe jailbreak: filtered: False detected: False custom_blocklists: filtered: False details: [ ] prompt_index: 1 content_filter_result: sexual: filtered: False severity: safe violence: filtered: False severity: safe hate: filtered: False severity: safe self_harm: filtered: False severity: safe custom_blocklists: filtered: False details: [ ] prompt_index: 2 content_filter_result: sexual: filtered: False severity: safe violence: filtered: False severity: safe hate: filtered: False severity: safe self_harm: filtered: False severity: safe custom_blocklists: filtered: False details: [ ] ] system_fingerprint: fp_d54531d9eb usage: completion_tokens: 1144 prompt_tokens: 2598 total_tokens: 3742 Recipe parsed successfully RecipeReader processing time: 47225 ms. |
Here you can see that the protected material filter was triggered. You still get an OK response but you have to look at the returned results and parse through the filters to determine if anything was triggered. A quick way to know is to parse the returned json, I have observed that if a filter is triggered the json returned is truncated, thus it won’t parse.
Another discovery is that when you are using a model that doesn’t support image input you will get an error that looks something like this: Error copying content to stream.
1 2 3 4 5 6 7 8 9 | ] ] model: phi-3-vision-128k-instruct-2 max_tokens: 8096 temperature: 0.1 top_p: 0.01 Using AI at: https://bradyguychambers-recipe-cfjsl.eastus.inference.ml.azure.com/scoreopenai/deployments/phi-3-vision-128k-instruct-2/chat/completions?api-version=2024-08-01-preview Error while copying content to a stream. Unable to write data to the transport connection: An existing connection was forcibly closed by the remote host.. |
My next post I’ll walk through using the OpenAI SDK for C#. And ongoing work on handling content filtering. I also hope to have some insight on different models how the perform and compare. In the mean time you are welcome to poke around the github repository it’s no where near read for example sharing but you might find something useful there.
https://github.com/bradyguyc/RecipeReader
Add comment
Comments